Why the risk matrix must die
Apr 27, 2017 • Jorn Mineur
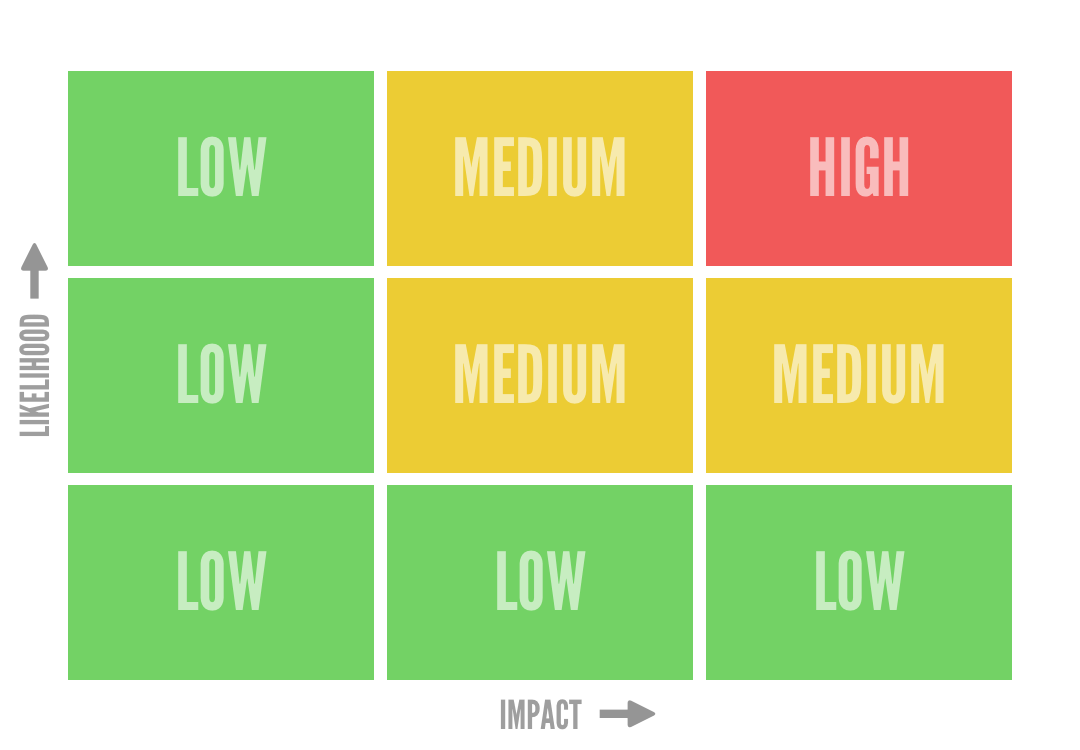
Risk assessment is at the heart of information security. The standard approach is to use a risk matrix to classify information security risks based on their probability and impact, then give each one a ‘risk score’ by adding the two numbers together. Then you rank the risks by score and address the top ones first.
The risk matrix is like an old, familiar friend. It’s been around for years and everyone uses it. It’s easy to understand and simple to use. And in terms of ‘getting to grips with risk,’ it seems to work.
There’s only one problem: it doesn’t.
I should know, because at E-accent we’ve been using the risk matrix ourselves for months. It’s baked into the information security management system (ISMS) we use every day. But I’ve come to realize that it’s actually unfit for purpose. In fact, it may even be doing more harm than good.
That’s pretty worrying when so many firms use this approach to manage big risks that could easily sink their business, and maybe their clients’ too.
If you’re one of those firms, read on.
Garbage in, garbage out
Things go wrong from the very beginning, with the probability estimates you put into the risk matrix.
On the whole, human beings are not very good with non-linear risks. Our instincts evolved to help us deal with immediate physical dangers in our environment. So we can tell whether an oncoming car is likely to hit us, for example. But the more complex the risk, and the more factors are involved, the less helpful our gut instinct is. And information security risks are some of the most fiendishly complex risks in the world.
It’s extremely difficult to say how likely it is that an information security incident will actually occur. So most people rely on gut instinct, on the grounds that it’s better than nothing.
But if you ask someone to gauge the likelihood of an information security risk — even someone with very deep knowledge — they will be hard pressed to give you an accurate answer. For instance, what’s the likelihood of a rogue ex-employee hacking your server? Is it low, medium or high? Why do you say that? How do you know?
It’s a similar story with impact. In theory, it’s easier to get a reasonably good idea of financial impact by thinking about management time, developer hours, lost sales and reputation damage. But people rarely bother, because the risk matrix is only asking for a simple assessment anyway.
Worse than useless
So the information you put into your risk matrix is hopelessly inaccurate. But then the matrix itself makes things even worse.
Because matrices they have such a low resolution, they make very different risks look alike. For example, in a 3x3 matrix (low, medium, high on both axes), risks with 67% probability and 99% probability are both ‘high.’ Clearly, you’d want to address the 99% risk first. But when you come to rank your risks, you have no way of knowing which one is worse based on the matrix.
What’s more, the matrix gives equal weight to probability and impact, so an incident with 1% probability and $200,000 impact has the same priority as one with 0.2% probability and $1,000,000 impact.
In fact, in some fairly common situations (mathematically speaking, when probability and impact are negatively correlated), you’d actually be better off choosing the matrix square at random. Yes, you read that right — pin your matrix to the wall, throw a dart for each risk and you’ve got a better chance of picking up the most important ones. The risk matrix can be, quite literally, worse than useless.
ISO27001 is all about learning and ongoing improvement. You have to show that the likelihood or impact of risks have gone down as a result of your efforts. But with the risk matrix, your measures are qualitative, subjective, inconsistent and uncalibrated in the first place, and they never get any more accurate over time. So it’s impossible to test whether anything has really changed, and it’s hard to justify investments in controlling risks.
Illusion of control
The problem with the risk matrix is that it feels scientific. It has what Stephen Colbert calls ‘truthiness’ — a plausible air of being true. It promises a quick, simple solution to a wicked problem without taking up loads of time, or asking you to do too many hard sums.
Before, you had no idea about risks. But now, you’ve put them in neat little boxes and given them solid-sounding scores. You’ve ‘got to grips’ with risk, or so it seems. But all you’ve really done is spin a fantasy that gives you a dangerous illusion of control.
It wouldn’t be so bad if that was the end of the story. But the risk matrix is the foundation of almost all risk assessment in information security, and is recommended by security advisory committees like NIST and OWASP. Your shonky guesstimates will ultimately determine how you prioritize risks, use resources and plan for disaster. But what’s the point of fortifying your castle if it’s built on sand?
When you don’t understand your taxes, you call your accountant. So why not call a risk management consultant? Because most of them are using this exact same method. You might feel reassured by involving an ‘expert,’ but all you’ve done is move the wrongness from your desk to theirs — and paid for the privilege.
If you have an information security strategy, you’re probably feeling pretty scared by now. I certainly was.
So I searched the web for hard evidence that risk matrices actually help manage risk. Was it possible that the whole thing was just snake oil?
What I found out was sobering. Not only is there no proof that risk matrices work, there’s actually proof of the opposite. Using the matrix actively hampers firms’ efforts to deal with risk, absorbing time, money and effort for no benefit at all.
The Bayesian way
What I did find, through the work of authors like Douglas Hubbard, was a better way to gauge probability. It’s called Bayesian statistics, and it’s been around for centuries.
Insurers, whose livelihoods depend on knowing probabilities as accurately as possible, use it all the time. For some reason, even though we’re in the business of “insuring” our data, it hasn’t penetrated the information security world. But it needs to.
The Bayesian approach uses hypotheses based on little or no data, which gradually become more accurate as you learn more. Don’t know how likely an event is? No problem. Start with related stuff that you do know something about, and work with it to sharpen up your probability estimate.
Traditional approaches to probability are frequentist: based on very large quantities of data about events in the past. But that’s not much use in information security, where you’re concerned with future risks that may never have happened yet, but could have very big impacts when they do. If you wait until then to gather your information, it could be too late to do anything.
Hubbard also looks at the unavoidable biases that come in when you use gut feeling. Instead, he suggests using percentages for probability (0%= impossible, 100% = certain) and dollars for impact.
From hunches to accuracy
Let’s see how it works in practice. For example, what is the risk that a server under your control will be hacked in the next 12 months? If you have no idea whatsoever, you begin by stating it as 50%.
Now, to sharpen up your figure, turn the question round and ask how you might be hacked. Like a detective, you need to consider means, motive and opportunity for different suspects. A foreign state? Unlikely for most businesses. An ex-employee with a grudge? More likely.
You still start with hunches, or whatever historical data you can get, and move forward from there. Heard about a new vulnerability in OpenSSL that allows hackers to hack your server in seconds? Your risk of data theft just skyrocketed.
You can do the same for impact. Instead of trying to gauge the total cost of an event, break it down into, say, legal fees, fines, developer time, reputational damage, lost sales and so on. All these things can be researched and made more accurate over time, which makes the amounts far more objective and accurate.
Since you’re bringing multiple probabilities together, it might seem that you’re multiplying the uncertainty. But the beauty of Bayesian statistics is that they actually cancel each other out. The more data you add, the more accurate your final probability estimate becomes. It’s like magic.
Now, the Bayesian approach is hard work. It takes longer, requires more effort and requires you to keep revisiting what you think about risk. But do you want the easy answer, or the right one?
In a world where cybersecurity risks are proliferating and getting worse, I don’t think we can afford to do anything else. The situation is clear: if we want our businesses to live, the risk matrix has to die.